Cognitive biases apply to most of us
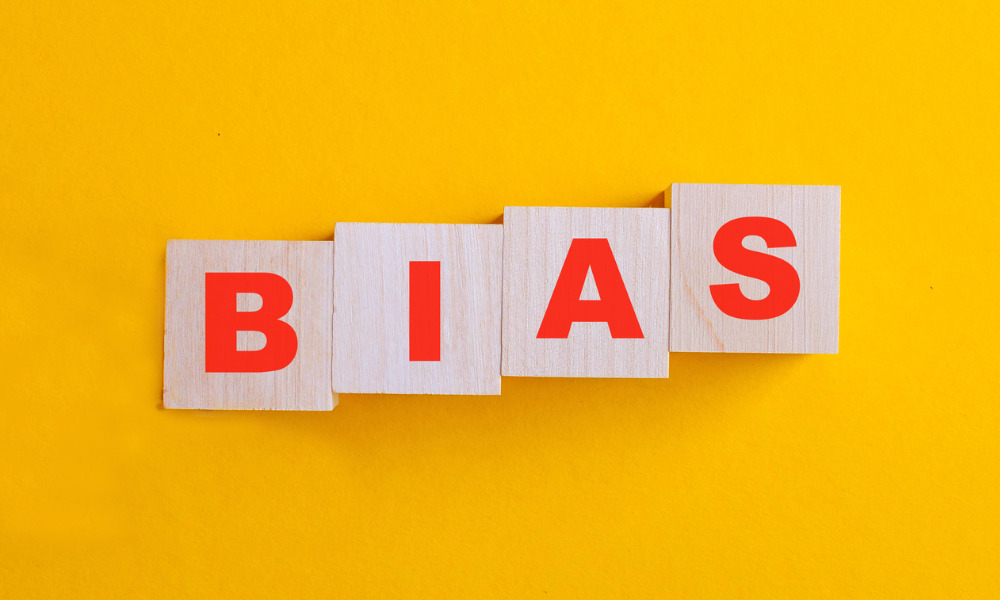
With the global pandemic, the word bias might be associated with mask making – but this article has nothing to do with bias tape. Global protests erupted with the killing of George Floyd, which does have to do with bias. There are different types of bias – cognitive biases (which are principally based upon studies of Western undergraduate students), as well as implicit biases.
Biases normally come in when we are judging something. Judging can be seen as a form of measurement. Before delving into measurement, we need to understand what bias really is.
Cognitive biases apply to most of us. As such, they are not shameful to possess. Drew Rae talked of hindsight bias1:
“Hindsight bias isn't just an accusation you throw to criticize someone who is looking at an event that's already happened. Hindsight bias is a universal psychological phenomenon. We all experience it. There's no escaping it. Everyone has hindsight bias. This was known about for quite a while before anyone gave it a name.”
There are places to learn about these nearly universal types of bias2. There are many of these, and being aware of them can help us be more cognisant of their effects on our thinking. Implicit bias is an inevitable part of being human. As mentioned by David Provan3:
“People might understand it as researcher bias... Like I said earlier, sometimes unconscious understanding of a person's own beliefs, they're not things that we always readily have available in front of our mind.”
The fact that many biases are not often at the front of our mind means that they can be working in our minds’ background, and we are not even aware of it.
An interview4 with the head of the RCMP reveals much about implicit biases:
“Rosemary Barton: ... do you believe there is systemic racism in the police system, including yours, in the country?
Brenda Lucki: That is an interesting question because in the last couple of days I have honestly heard about 15 or 20 different definitions of systemic racism and if it refers to an unconscious bias that exists, we have that in the RCMP. And we are not immune to it at all, in any case.
Immunity from implicit bias is not possible.”
Unacknowledged bias, though, can have real consequences:
“A bias in health care can result in a positive or negative disposition towards a patient based on ethnicity, gender, age, socio-economic status, looks, charisma, common interests, etc. When you like someone you may be more likely to want to accede to his or her request. This can be a normal reaction, but one that physicians need to be aware of and resist.5”
Learning of one’s implicit biases can be a challenging exercise of self-discovery. Sometimes, our close contacts will be kind enough to tell us some of our implicit biases. LinkedIn has a course on implicit bias6, as well as the Harvard Implicit Bias tests7. These tests come highly recommended, although test takers need to be ready to face the results. There are also some excellent posts on LinkedIn regarding implicit bias8.
So, where does this leave us?
One possibility is to return to the idea of judgement as being a measurement. Measurements are imprecise representations of reality. Physical sciences expect that measurements are accompanied by their uncertainties, to enable the receiver of the measurement to know how much to trust it. When I was a graduate student, I marked 1st year student’s labs (in part) on the uncertainty (error) associated with any measurement.
Total uncertainty = SQRT(Systematic error^2 + Random error^2)
Systematic error is usually given by the manufacturer of the measuring instrument. The random error is repeating the measurement multiple times and calculating the spread of the measurements. Overall, any physical measurement should be accompanied by an associated uncertainty.
The idea is not to deny that there is uncertainty, or to do everything possible to make it go away, but to make it transparent, so that the accuracy of the measurement can be judged accordingly. I think this can be helpful in the treatment of bias.
Knowing what biases we have (cognitive and implicit) is a formidable task, and one that helps us on our own individual improvement journeys. These would form the equivalent of the systematic error in the physical measurement.
Regarding the random error, and the wisdom of crowds, Choiceology9 had a great suggestion to harness “the crowd within”:
“...on one day, our judgments aren’t that well correlated with our estimates on another day….So it was helpful to inject more noise into people’s guesses by giving them time to forget their initial estimates and come up with a truly distinct new opinion.”
So, instead of trying to render our decisions and our thinking completely unbiased (which I believe is an impossible task), why don’t we learn more about our biases, and then try to be transparent with them when making judgements.
For example, a manager is hiring for a position. Some ways to address bias might include:
- Structurally: Ensuring bias susceptible information (e.g. name, race, neighbourhood) are blind to the manager
- Implicit: Manager takes implicit bias tests, and is aware of the likely bias she brings to a decision on a certain demographic
- Cognitive: Manager learns the most likely decision making cognitive biases (e.g. anchoring bias - the first candidate being the point of comparison, framing effect - presentation of information affecting its comprehension, etc.)
- Random: The manager can rate the candidates at different times (on resumes, after interview, weeks thereafter) and average these rates per candidate
Then, when deciding on a candidate, all the biases above can be explicit, showing how confident the manager should be in selecting a candidate.
If biases are treated analogously to errors in physical measurements, we may be in a better place to know how much they are influencing our thinking. We can work on trying to reduce them, but total elimination is not possible. Transparent reporting of them, however, should be.
If you would like to talk more about biases, let’s chat [email protected], or message me on LinkedIn.
Footnotes:
1. https://safetyofwork.com/episodes/ep-21-how-foreseeable-was-the-dreamworld-accident/transcript
2. Wikipedia has a decent list and references on its “List of cognitive biases” page
3. https://safetyofwork.com/episodes/ep30-what-do-safety-professionals-believe-about-themselves/transcript
4. https://www.youtube.com/watch?v=yQsapPBHefY
5. https://health.gov/our-work/health-care-quality/trainings-resources/pathways-safer-opioid-use/training
6. https://www.linkedin.com/feed/news/combating-bias-at-work-learn-more-4155929/
7. https://implicit.harvard.edu/implicit/takeatest.html
8. For example, look at https://www.linkedin.com/in/amaechi/
9. https://www.schwab.com/resource-center/insights/content/choiceology-season-5-episode-1