How to break the blame cycle
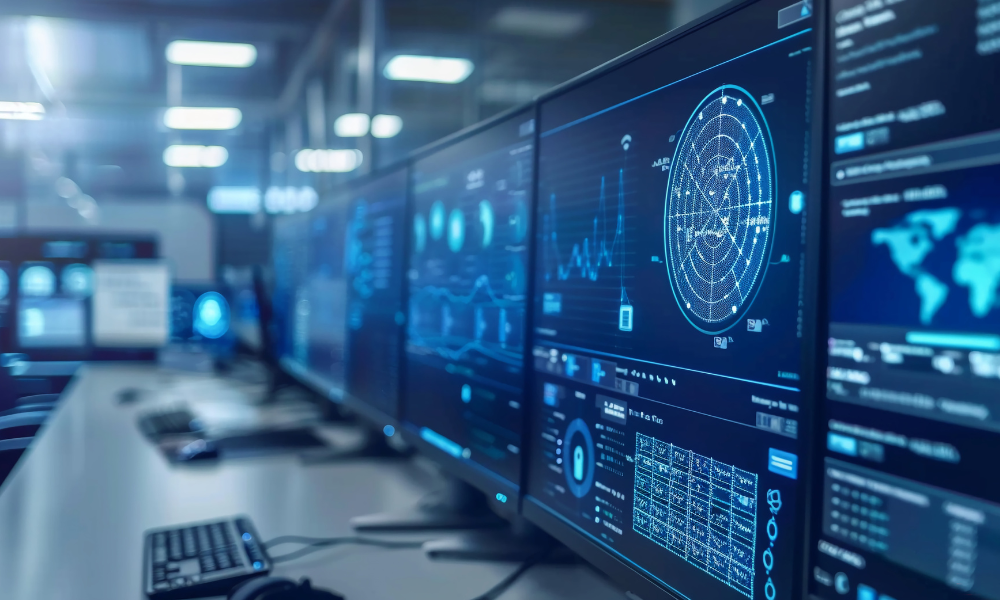
Introduction
In many manufacturing plants we consult for, safety incidents were handled with an all-too-familiar response: find the person closest to the incident, determine what they did wrong, and retrain them. The leadership team weren't bad people—they genuinely wanted to prevent recurrences—but their approach fostered a culture of fear, where workers began concealing close calls and other events, only for similar incidents to reemerge months later.
This scenario plays out in organizations worldwide. Safety experts call this the "name, blame, shame, and retrain" cycle. These approaches typically focus on identifying individual errors rather than understanding the complex systems in which they occur.
"Underneath every simple, obvious story about 'human error,' there is a deeper, more complex story about the organization" (Dekker, 2014).
This is where generative AI can help. Not to replace human investigators, but to work alongside them as a thought partner. It brings powerful new tools to significantly boost objectivity, thoroughness, and effectiveness in incident investigations. Generative AI represents a seismic shift in how we approach learning from incidents. AI’s power lies in its ability to bring objectivity, multiple data sources and insights from multiple sources to help us see a bigger picture.
The Current State of Incident Analysis and Investigation
Traditional incident investigation approaches face several critical limitations:
- Confirmation bias: Investigators often look for evidence that confirms their initial assumptions about what happened.
- Hindsight bias: After an incident occurs, it's easy to see what went wrong and judge decisions made in the moment by what we know now.
- Fundamental attribution error: We tend to attribute others' actions to their personal characteristics rather than situational factors.
- Administrative focus: Organizations frequently implement additional rules, procedures, and training rather than addressing engineering controls or system design issues.
- Documentation challenges: Updating procedures and related materials following incidents is often slow, inconsistent, and incomplete.
Fundamental Applications of Generative AI in Incident Analysis
Generative AI offers several groundbreaking capabilities that can transform incident investigation:
AI can assist across the entire incident analysis process, structured in distinct but interrelated phases:
- Preparation: Supporting the creation of a clear problem statement, outlining incident investigation team roles, and preserving original evidence.
- Question Development: Generating open-ended, non-leading questions that focus on system interactions rather than individual fault.
- Interview Support: Recording and analyzing interviews to capture the entirety of the conversation, gauge tone and sentiment, and help identify overlaps or inconsistencies in witness accounts.
- Data Gathering and Integration: Pulling and synthesizing information from video footage, audio logs, equipment photos, procedures, permits, interviews, and more.
- Pattern Recognition and Data Analysis: Identifying trends across data sets, linking contributing factors, and highlighting systemic contributors such as production pressures or design flaws.
- Procedure and Usability Evaluation: Assessing how practical and accessible procedures were in the context of real work conditions.
- Recommendations Development: Generating actionable insights focused on system redesign, engineering controls, and leadership and culture gaps, while reducing reliance on administrative fixes.
- Reporting and Learning Loop Integration: Assisting in the creation of clear, bias-free reports and embedding findings into organizational learning systems including procedures, audit forms and training materials.
Multi-Format Analysis Capabilities
Unlike human investigators who may struggle to integrate diverse information sources, AI can simultaneously process:
- Audio recordings of witness interviews
- Video footage from before, during, and after incidents
- Photographs of equipment and incident scenes
- Technical documentation including blueprints and P&IDs
- Written procedures and work permits
- Historical incident data and near-misses
This integration capability allows for more comprehensive analysis and can reveal connections that might otherwise be missed.
In organizations that have implemented AI-enhanced investigations, workers often feel relief. Many express that they feel the investigation is focused on understanding the complete picture rather than assigning blame. This shift enables honest conversations about how work actually happens, including the pressures, constraints, and daily adaptations that traditional approaches often miss.
Systems-Level Applications
A valuable application of AI in incident investigation is its ability to identify contributing factors beyond individual actions. Traditional investigations often stop at the "human error" level, but AI can help organizations:
- Map complex system interactions: Visualizing how decisions at different organizational levels influenced the incident.
- Identify latent organizational issues: Recognizing patterns that suggest deeper systemic problems.
- Analyze production pressure: Quantifying how schedule demands may have contributed to risk-taking.
- Evaluate procedure usability: Assessing whether procedures were workable in real-world conditions.
As James Reason, creator of the "Swiss Cheese Model" of accident causation, emphasizes: "Rather than being the main instigators of an accident, operators tend to be the inheritors of system defects created by poor design, incorrect installation, faulty maintenance and bad management decisions. Their part is usually that of adding the final garnish to a lethal brew whose ingredients have already been long in the cooking" (Reason, 1990).
AI can help identify those "system defects" and trace the "ingredients" that contributed to the incident, offering a more complete understanding than traditional methods typically provide.
Challenging Assumptions and Overcoming Bias with AI
One of the most transformative potentials of generative AI lies in its capacity to challenge our deeply held assumptions and reduce the cognitive biases that often compromise the quality of incident investigations. Biases such as confirmation bias, hindsight bias, and the fundamental attribution error are not just occasional glitches in thinking; they are ingrained human tendencies that can mislead investigations and obscure systemic issues.
Generative AI mitigates these risks in several ways:
- Objective analysis: AI doesn't bring assumptions, past experiences, or politics into the investigation. This allows it to surface patterns and factors that may go unnoticed by humans with preconceived narratives.
- Consistent logic: AI follows a clear reasoning process based on data, helping remove emotional judgments or managerial pressure to "find fault" quickly.
- Counterfactual exploration: Advanced models can simulate alternative scenarios or explore what might have happened under different conditions, broadening the lens through which causes are examined.
- Pattern disruption: AI can flag when investigators are repeating past conclusions, encouraging a step back to consider fresh possibilities.
- Language auditing: It can analyze the phrasing used in interviews, reports, or even policies to detect blame-laden or biased framing that may influence outcomes.
- Critical feedback loops: Perhaps most importantly, investigators can and should intentionally prompt AI to challenge their preliminary and final conclusions. This deliberate questioning—asking the model to play devil's advocate or propose alternative interpretations—can highlight blind spots and force reconsideration of assumed facts or narrow diagnoses.
By confronting our mental shortcuts and institutionalized habits, AI becomes a partner in critical thinking, helping us investigate not only the incident, but how we think about the incident.
A most profound benefit of AI-enhanced investigations is how they contribute to improved worker trust. When employees see that incidents are treated as learning opportunities rather than occasions for blame, they become more willing to speak openly about risks, concerns, and close calls. This transparency creates a virtuous cycle: better information leads to more effective prevention, which builds trust and encourages further openness. The result is a culture where safety becomes a collective commitment rather than a compliance exercise.
Elevating Traditional Root Cause Analysis Tools
Returning to our manufacturing plant example, we found that AI didn't just introduce new methods, it transformed our existing approaches. Two traditional tools in particular gained new depth through AI integration: Why Analysis and Barrier Analysis.
Why Analysis: Breaking Beyond Linear Thinking
Traditional "5 Whys" approaches often follow a single path of questioning that conveniently stops at human error, leading predictably to more training or procedures. In our plant in question, integrating AI transformed this process by exploring multiple lines of questioning simultaneously, revealing system patterns that remained hidden in conventional approaches.
During a quality deviation investigation, rather than stopping at "the operator wasn't properly trained," our AI partner opened parallel paths of inquiry: Why was the procedure written ambiguously? Why did the interface design contribute to errors? Why had similar patterns occurred with different operators? This revealed that frequent procedure revisions, misaligned terminology, and rushed shift handovers had created a perfect storm of conditions where errors became likely, if not inevitable.
Barrier Analysis: Seeing Systems, Not Just Gaps
When examining protective barriers, traditional approaches focus narrowly on which specific safeguard failed. With AI assistance, we began mapping relationships between physical, procedural, and cultural barriers, revealing how weaknesses in one area created pressures on others.
In one near-miss involving incomplete lockout/tagout, our enhanced approach uncovered not just a procedural gap but a system where verification was cumbersome, supervision was stretched thin, and an informal culture had developed where experienced workers managed safety through workarounds rather than following increasingly complex procedures. This systems view led to redesigned processes that were both more protective and more practical in real-world conditions.
As the maintenance manager observed, "We've been adding more and more rules without understanding why people work around them. Now we're finally seeing the whole system instead of just the holes in it."
The Human-AI Partnership: Complementary Strengths
While AI brings powerful analytical capabilities to incident investigation, the human element remains irreplaceable. The most effective approach is a thoughtful partnership that leverages the unique strengths of both:
- AI strengths: Data processing at scale, pattern recognition, bias reduction, consistency, and tireless analysis of complex information.
- Human strengths: Contextual understanding, empathy, ethical judgment, creative thinking, and the ability to build trust with those involved in the incident.
This partnership works when human investigators maintain their role as thought leaders—guiding the inquiry, interpreting findings through their experiential wisdom, and making value-based judgments about what matters most. AI serves as an amplifier of human capabilities rather than a replacement, providing insights and challenging assumptions while humans maintain ultimate responsibility for conclusions and recommendations.
Practical Next Steps for Organizations
Organizations interested in integrating AI into their incident investigation processes can start with these practical steps:
- Start small: Begin with focused applications while building confidence in the approach. Use AI to analyze the language in historical incident reports to identify where bias may have influenced conclusions. Have AI generate open-ended interview questions for your next investigation. AI can also be used to assess practical usability of procedures against real-world conditions.
- Build capacity: Create opportunities for investigation teams to practice with AI tools in low-stakes scenarios before applying them to significant incidents.
- Address resistance thoughtfully: Acknowledge concerns from safety professionals who may worry about technology diminishing their role. Position AI as an amplifier of human wisdom rather than a replacement.
- Establish ethical guidelines: Create protocols for data privacy and use of AI insights.
- Measure effectiveness: Track how AI integration affects investigation quality and recommendation implementation.
- Streamline document revision: Use AI to verify that procedures, audit guidance and training documents are updated based upon investigation findings.
- Create feedback loops: Continuously improve AI applications based on user experience and investigation outcomes.
Conclusion: From Blame to Learning
Remember our manufacturing plant story? Six months after implementing an AI-enhanced investigation approach focused on systemic understanding and reliability rather than individual blame, something remarkable happened. A near-miss occurred when a maintenance technician almost contacted an energized circuit. In the past, this might have resulted in a quick reprimand and retraining on lockout-tagout procedures.
Instead, the new investigation process revealed a broader picture. The AI-assisted analysis identified patterns across multiple data sources: the technician was working near the end of a 12-hour shift; three critical procedures had been updated in different systems creating confusion; the lighting in the area had degraded over time; and similar near-misses had occurred but hadn't been connected. Most importantly, the investigation revealed that the technician had improvised a safety measure that prevented what could have been a fatal incident.
Rather than punishment, the outcome was a redesign of the electrical system with improved isolation capabilities, consolidated procedures with better visual aids, improved lighting standards, and a recognition program for workers who identified safety improvements. The technician became an advocate for the new approach, telling colleagues, "For the first time, I felt like they actually wanted to understand what really happens out here instead of just finding someone to blame."
Reference List
- Dekker, S. (2014). The Field Guide to Understanding 'Human Error'. Ashgate Publishing.
- Conklin, T. (2019). Pre-Accident Investigations: Better Questions – An Applied Approach to Operational Learning. CRC Press.
- Reason, J. (1990). Human Error. Cambridge University Press.
- Hollnagel, E. (2014). Safety-I and Safety-II: The Past and Future of Safety Management. Ashgate Publishing.